Enhancing Decision-Making with AI-Powered Predictive Analytics Services
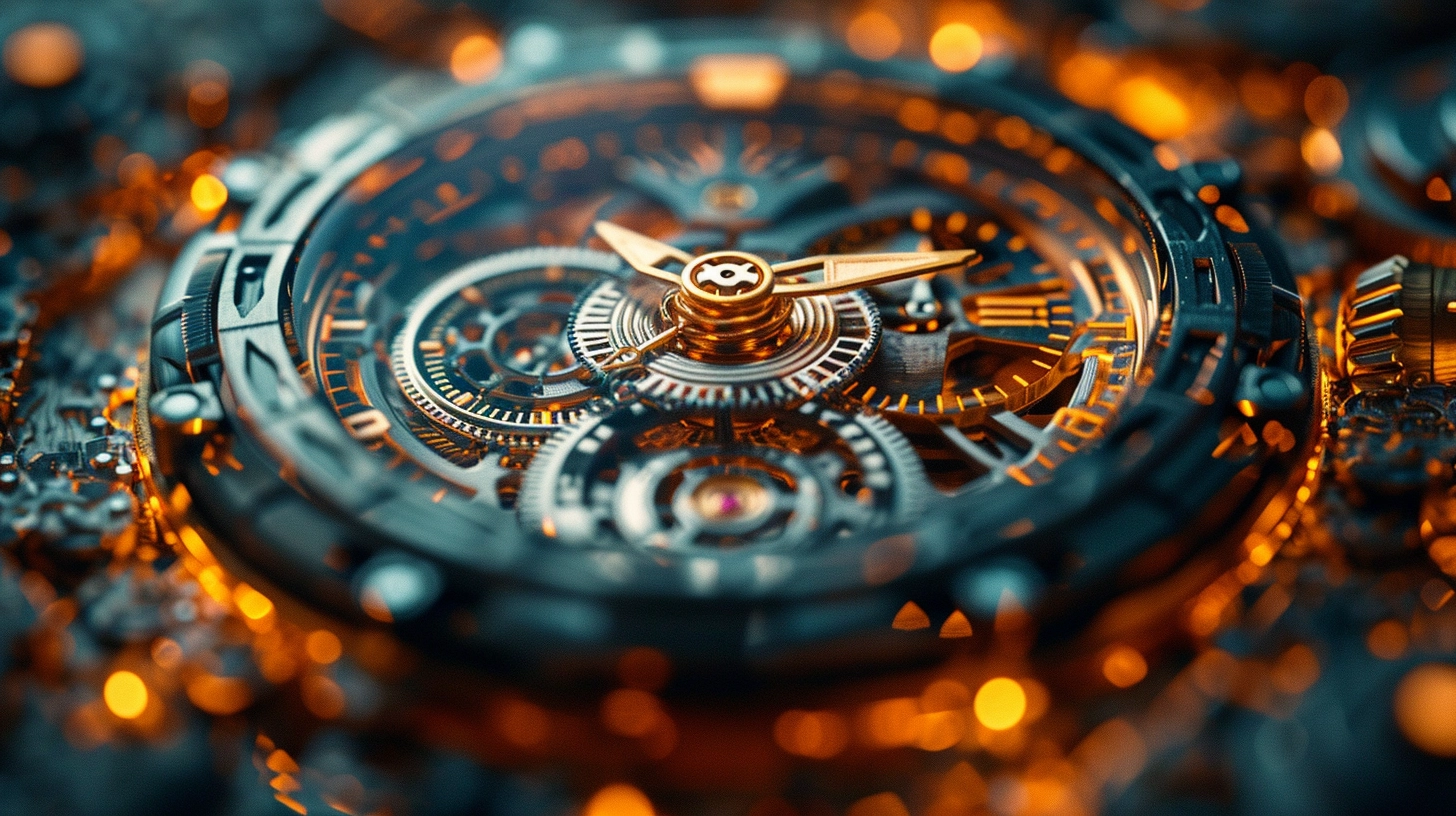
Enterprises struggle to make data-driven choices and unlock advantageous strategies due to the complexity of data and analytics.
Despite the necessity of machine learning algorithms, past data analysis, and data science, companies often find it challenging to enhance decision-making and predict future patterns effectively.
The trends for AI in predictive analytics services mark a significant leap in AI integration across products and services. This integration is revolutionizing the data and analytics life cycle, offering augmented tools for data quality checks, data cleansing, and advanced analytics features.
It streamlines processes, enhances data management efficiency, and unlocks new insights and automation capabilities across various industries.
Key Takeaways
- AI-powered predictive analytics services optimize decision-making and forecasting trends for strategic advantage.
- Integrating AI into products enhances the efficiency of data management and brings new insights and capabilities.
- Generative AI will shift towards using organizational data, allowing for more personalized and customized solutions.
- The evolution of AI’s infrastructure leads to better performance, scalability, and storage efficiency.
- Advancements in AI tuning mechanisms enable more context-specific adjustments and relevance in diverse business contexts.
Ready to predict the future of your business?
Use this prompt to tailor AI predictive analytics services to your specific business needs.
AI predictive analytics services harness advanced algorithms and machine learning techniques to analyze data and forecast future trends, behaviors, and outcomes. These services are invaluable across various sectors, including finance, healthcare, retail, and more, enabling businesses to make informed decisions, optimize operations, and enhance customer experiences. Here's a customizable prompt to help you explore the development or enhancement of AI predictive analytics services for specific business needs:
### Customizable Prompt for Exploring AI Predictive Analytics Services
"How can [Company Name], operating in the [Industry], leverage AI predictive analytics services to forecast [Specific Business Outcomes, e.g., customer behavior, sales trends, operational risks]? Consider the integration of machine learning models, data mining techniques, and real-time analytics to improve decision-making and strategic planning."
#### Areas to Explore:
1. **Identifying Data Sources**: What key data sources should [Company Name] focus on to feed the predictive analytics models? Consider internal data, public datasets, and real-time data streams relevant to [Industry].
2. **Model Development and Training**: How can [Company Name] develop and train accurate and reliable predictive models tailored to forecasting [Specific Business Outcomes]? Discuss the selection of algorithms, feature engineering, and validation methods.
3. **Integration into Business Processes**: In what ways can the predictive analytics services be integrated into the existing business processes of [Company Name]? Explore the potential for automating decisions, enhancing customer experiences, and optimizing operations.
4. **Ethical Considerations and Bias Mitigation**: What ethical considerations should [Company Name] keep in mind when deploying AI predictive analytics? How can the company ensure the responsible use of data and mitigate biases in predictive models?
5. **Performance Monitoring and Model Updating**: Discuss the strategies for continuously monitoring the performance of predictive analytics services and updating models based on new data and feedback to maintain accuracy over time.
6. **Exploring Use Cases**: Identify specific use cases within [Industry] where AI predictive analytics could provide significant value to [Company Name], such as demand forecasting, risk assessment, or personalized marketing strategies.
### Guide for Use:
- Personalize the prompt with your company's name, the industry you operate in, and the specific outcomes you wish to predict using AI predictive analytics.
- Use the insights generated to identify potential data sources, develop a roadmap for model development, and integrate predictive analytics into business operations.
- Consider consulting with AI and data science experts to ensure the effective implementation of predictive analytics services, especially in complex or highly regulated industries.
- Stay informed about advancements in AI technologies and predictive analytics methodologies to continuously refine and enhance your services.
Leveraging AI predictive analytics allows businesses to not only anticipate future trends and behaviors but also to proactively address challenges and seize opportunities, driving innovation and competitive advantage.
The Trend of AI in Product Integration
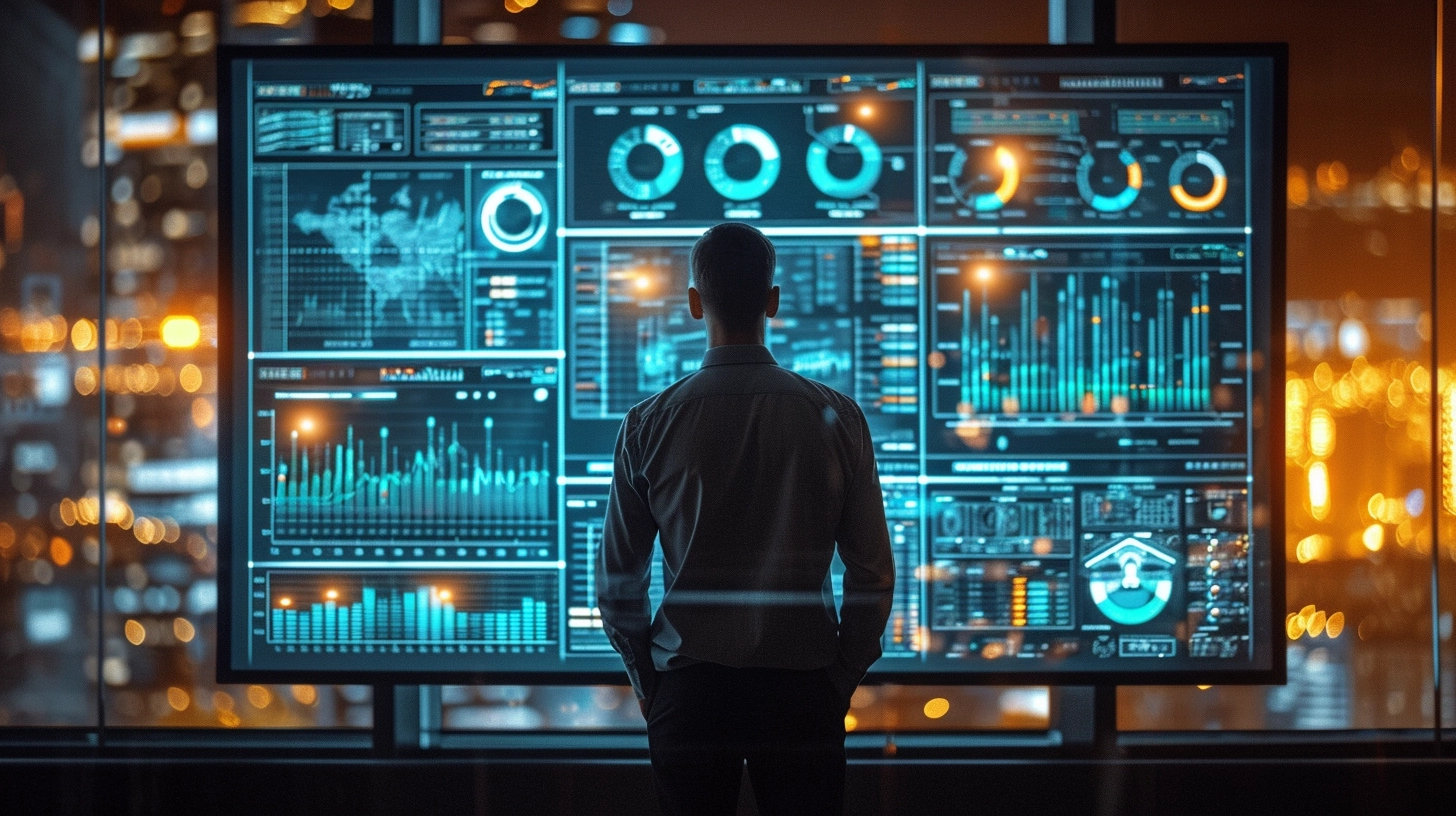
Today, the tech world is buzzing with the latest trend: AI integration in products, transforming the data and analytics life cycle. How is this trend reshaping businesses?
AI-Powered Augmented Tools Enhancing Data Quality
AI-Augmented Tools for Data Quality and Cleansing
AI-augmented tools are revolutionizing the way businesses handle data quality. These tools not only conduct data quality checks but also automate data cleansing. This is a significant development considering that businesses traditionally spend numerous hours correcting data errors. With AI algorithms efficiently detecting and fixing data errors, there is a substantial saving in time and resources.
Key Benefits:
- Automated Data Cleansing: AI swiftly identifies and rectifies data errors.
- Reliable Data for Decision Making: Enhanced data quality leads to more confident and informed business decisions.
Generative AI in BI Tools Transforming Analytics
Revolutionizing Predictive Analytics with Generative AI
The integration of generative AI into business intelligence (BI) tools marks a pivotal change. It acts like a virtual analyst, capable of creating data that mirrors real-world scenarios. This greatly enhances predictive analytics, offering more realistic data models for improved forecasting and planning.
User-Friendly BI Tools with Natural Language Interfaces
A key feature of this integration is the inclusion of natural language interfaces in BI tools. This makes these tools vastly more accessible and easy to use, allowing users to interact with data through simple language. This feature is particularly beneficial for those without technical expertise, broadening the user base.
Advantages of Natural Language Interfaces:
- Ease of Use: Simple, intuitive engagement with data.
- Improved Collaboration: More effective communication of insights across diverse teams.
The Future is Now: AI-Powered Products Driving Business Growth
Based on the research, the integration of AI in products is not just a trend; it’s the future. Here’s why:
- Streamlined Processes: AI automates and simplifies complex tasks, boosting efficiency.
- Augmented Decision Making: AI provides deeper insights, enhancing human decision-making.
- Innovation and Growth: New AI capabilities open doors to untapped opportunities and innovation.
AI Integration in Products | Impact on Business |
---|---|
Augmented tools for data quality | Improved efficiency and accuracy |
Generative AI in BI tools | Enhanced predictive analytics and user experience |
Natural language interfaces | Simplified data interaction and better collaboration |
According to statistics, companies leveraging AI in their products witness significant growth in efficiency and innovation.
The integration of AI in products is reshaping the business landscape. By embracing these advancements, companies can unlock new potentials, streamline operations, and soar to new heights of success. As AI evolves, its integration into products will continue to be a critical factor in driving business success and innovation.
The Shift Towards Using Organizational Data in Generative AI
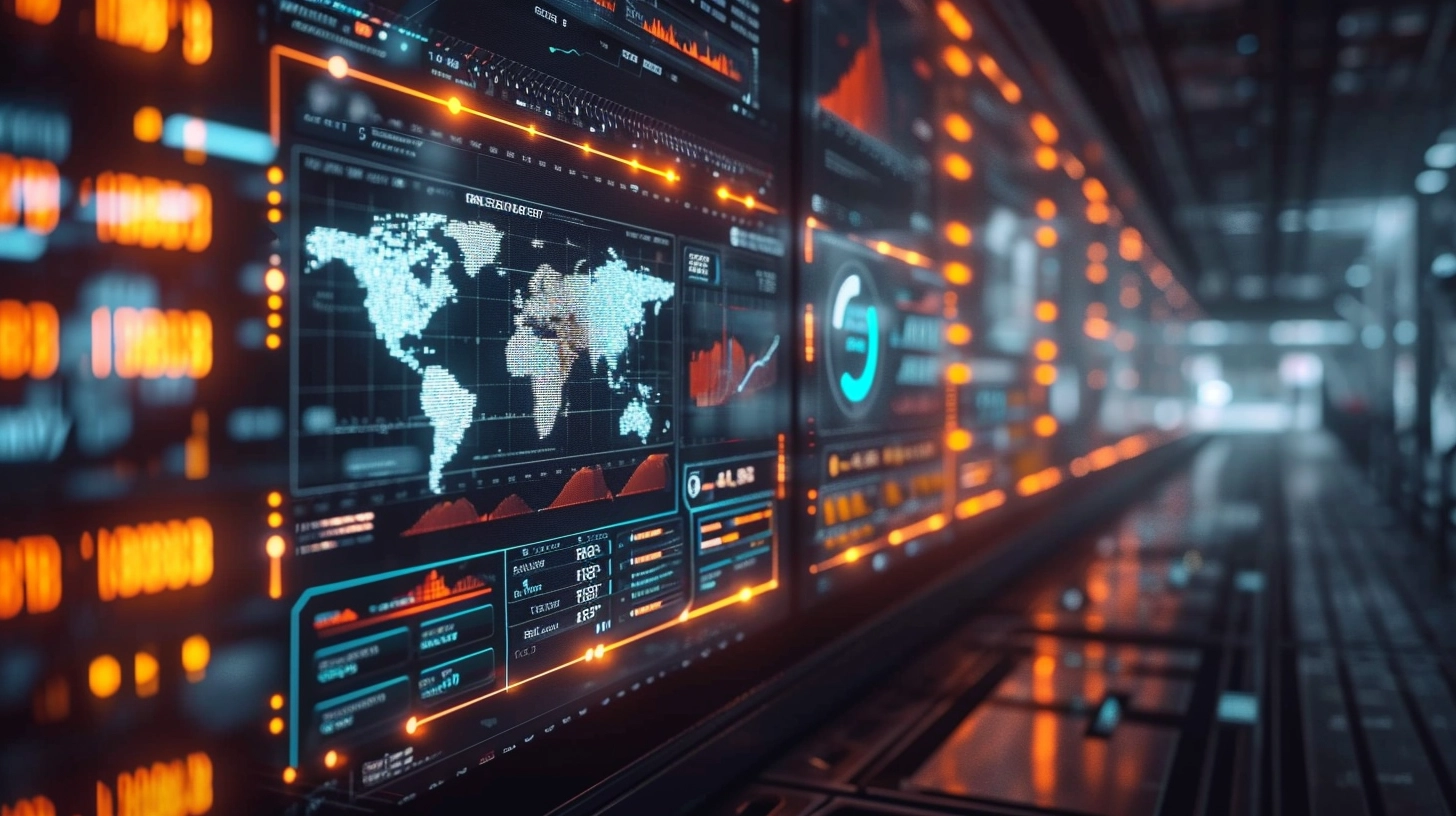
In recent years, businesses have been undergoing a significant shift in the way they utilize generative AI, with a strong focus on harnessing their own organizational data. This shift enables companies to leverage their proprietary data to create customized AI applications tailored to their specific needs, while also ensuring the security and confidentiality of sensitive data.
By hosting private foundation models within their own infrastructure, businesses can maintain control over their data, maximizing the potential of generative AI for customized solutions.
This trend paves the way for innovative applications of generative AI across various business functions. For example, customer support chatbots can be trained using company-specific data, enabling them to deliver more personalized and effective assistance to customers.
Marketing departments can leverage generative AI to create personalized messages for targeted marketing campaigns, utilizing customer data to deliver tailored content that resonates with individual preferences.
This shift towards using organizational data in generative AI unlocks new possibilities for businesses to derive insights and drive growth. By combining the power of AI with their proprietary data, companies can uncover patterns, trends, and correlations that may have otherwise gone unnoticed.
This allows for more informed decision-making, enabling businesses to stay ahead of the competition and deliver enhanced customer experiences.
Benefits of Using Organizational Data in Generative AI:
- Customized AI Applications: By leveraging organizational data, businesses can create custom AI applications that address their specific needs and challenges.
- Enhanced Customer Support: Customer support chatbots trained on company-specific data can provide personalized and efficient assistance to customers, improving overall customer satisfaction.
- Personalized Marketing Campaigns: Generative AI can be used to personalize marketing messages based on customer data, increasing engagement and conversion rates.
- Uncovering Insights: By combining generative AI with proprietary data, businesses can uncover valuable insights, patterns, and trends that drive informed decision-making.
The Evolution of AI’s Infrastructure
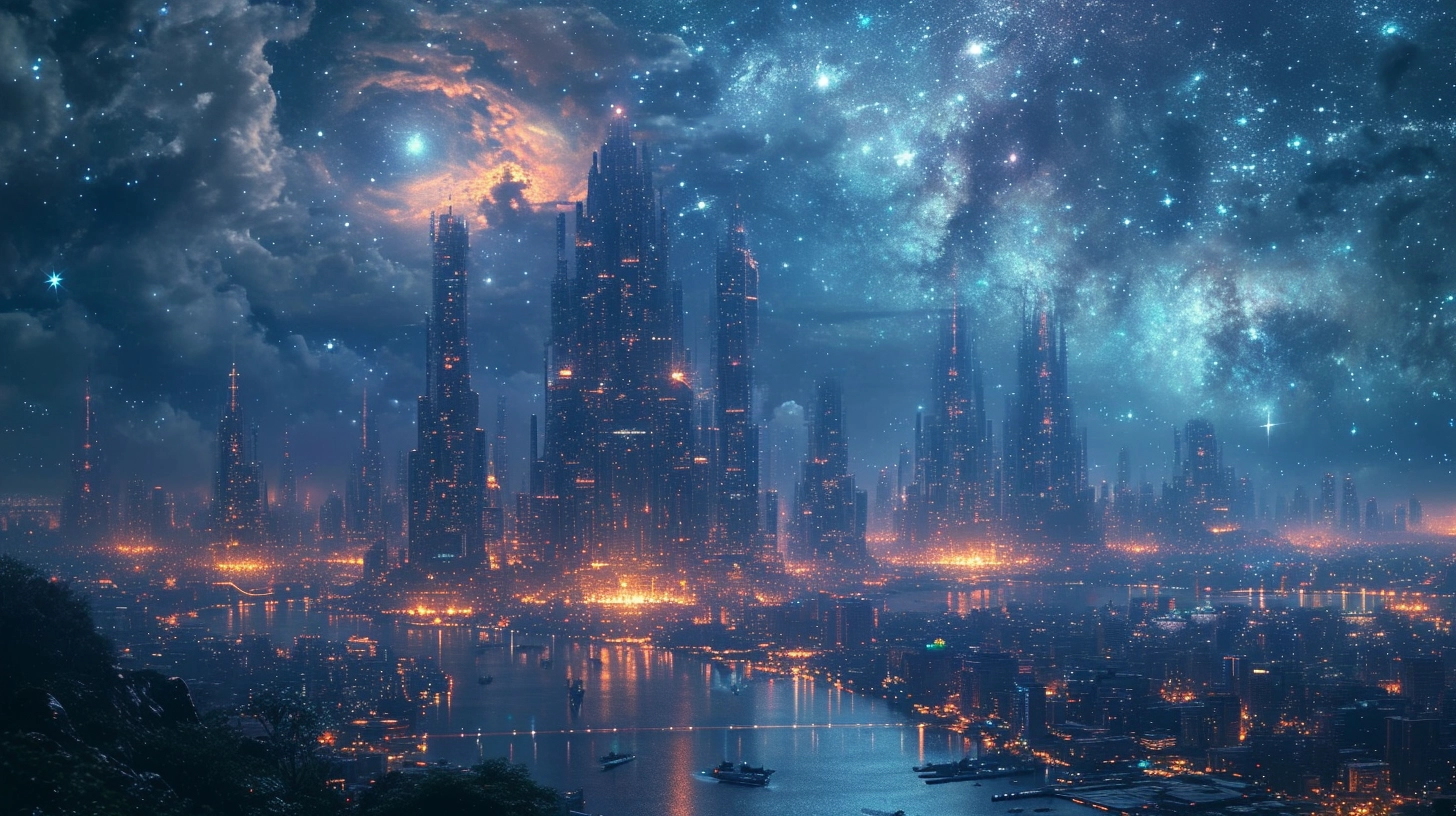
As AI continues to permeate various industries, the infrastructure supporting AI applications must evolve to meet the increasing demands. In 2024, businesses will focus on refining their architectural frameworks, particularly in the context of generative AI.
This evolution will involve the adoption of new database technologies, such as vector databases, which are specifically designed to manage the intricate data representations utilized by AI models. By embracing these changes, companies can achieve more efficient data processing, improved performance, and greater scalability.
One of the most significant advantages of updating AI infrastructure is the ability to store and retrieve data more efficiently. Traditional databases may struggle with the volume and complexity of data generated by AI applications, leading to performance bottlenecks.
On the other hand, vector databases are tailored to handle high-dimensional data and are optimized for efficient storage and retrieval, thereby enabling better performance and scalability.
Benefits of Evolving AI Infrastructure
By embracing the evolution of AI infrastructure, businesses can unlock various benefits:
- Enhanced Data Processing: Optimized data storage and retrieval enable faster and more efficient processing, facilitating real-time analysis and decision-making.
- Improved Performance: The use of vector databases and other advanced technologies can significantly enhance the performance of AI applications, allowing for quicker and more accurate results.
- Greater Scalability: Upgrading infrastructure empowers businesses to scale their AI capabilities without sacrificing performance or data integrity.
- Expanded Application Potential: Efficient data management enables the exploration of more complex AI models and novel applications that were previously unattainable.
Reevaluating Data Management Strategies
While evolving AI infrastructure promises enhanced performance and scalability, businesses must also reevaluate their data management strategies to fully capitalize on these advancements.
This reevaluation involves establishing efficient data pipelines, implementing robust data governance policies, and ensuring adequate data quality control measures.
By prioritizing effective data management, organizations can unleash the true potential of AI and drive meaningful insights for data-driven decision making.
Key Considerations for Evolving AI Infrastructure | Benefits |
---|---|
Prioritize the adoption of vector databases and other suitable technologies | Efficient data storage and retrieval Improved performance and scalability |
Establish streamlined data pipelines | Enhanced data processing Real-time analysis capabilities |
Implement robust data governance policies | Increased data integrity Improved compliance and security measures |
Invest in data quality control measures | Accuracy and reliability of AI models Data-driven decision making |
By embracing advancements in AI infrastructure, organizations can unleash the full potential of AI applications, enabling faster and more accurate data processing, better performance, and greater scalability.
This evolution requires businesses to adopt suitable architectural frameworks, leverage vector databases, and reevaluate data management strategies. By doing so, companies can harness the power of AI to gain a competitive edge and drive innovation in today’s data-driven world.
the Power of Prompt Tuning and RAG
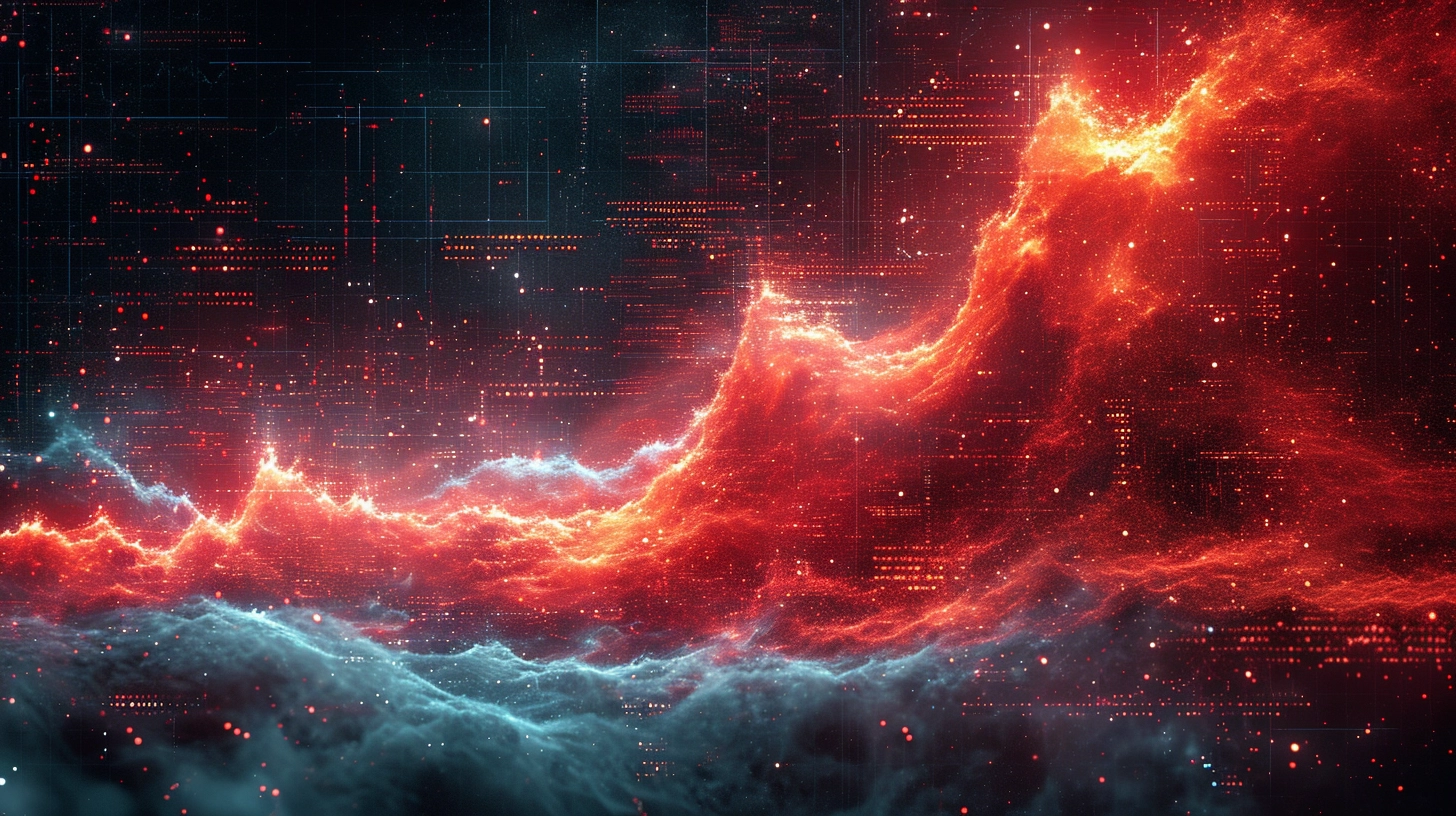
In the ever-evolving landscape of Artificial Intelligence, 2024 marks a significant leap with the introduction of prompt tuning and retrieval augmented generation (RAG). These novel tuning techniques are set to revolutionize the field of AI, offering more precise and context-specific adjustments to AI models.
Prompt Tuning: The Game Changer in Flexibility and Adaptability
Prompt tuning has emerged as a key player, utilizing smaller pre-trained models to encode text prompts. This advancement allows for incredible flexibility and adaptability in AI-driven outputs. Organizations can now swiftly tailor AI models to address current business scenarios, ensuring the system’s responses are perfectly aligned with user needs and preferences. This technique is a boon for businesses, offering the ability to provide prompt and highly contextual insights.
Benefits of Prompt Tuning in Business Contexts
- Customization: Tailors AI responses to specific user needs.
- Efficiency: Reduces the need for extensive retraining.
- Adaptability: Quickly adapts to changing business scenarios.
Retrieval Augmented Generation: Enhancing AI with Contextual Relevance
Retrieval augmented generation (RAG), on the other hand, is all about augmenting AI outputs with relevance and accuracy. By combining specific information from a knowledge source with prompts, RAG enhances the generation process. This ensures that AI systems produce more contextually appropriate responses, especially when dealing with complex and nuanced data sets.
RAG’s Impact on Data Handling
- Contextual Accuracy: Provides more relevant AI responses.
- Data Utilization: Leverages existing knowledge sources effectively.
- Insight Extraction: Helps extract meaningful insights from complex data.
Unleashing AI’s Full Potential in Business
These advancements in AI tuning mechanisms are unlocking new horizons for organizations to leverage AI technology. By making AI models more adaptable and effective, businesses are now equipped to harness AI’s power in diverse contexts. This not only addresses the challenges of computationally expensive AI training but also enables organizations to seamlessly adapt AI systems to diverse business scenarios.
Enhancing AI Models with Prompt Tuning and RAG
To better illustrate the impact of prompt tuning and retrieval augmented generation (RAG), let’s consider a scenario where a marketing manager is using an AI-powered system to generate personalized marketing campaigns. Using prompt tuning, the marketing manager can input specific parameters, such as target audience demographics, product features, and campaign objectives, and receive tailored marketing messages that resonate with their target customers.
The use of RAG further enhances the system’s ability to generate contextually appropriate marketing messages. By retrieving relevant data from the company’s proprietary databases, the AI system can augment its prompts with accurate and up-to-date information, ensuring that the marketing campaigns are highly relevant to the current business landscape and specific customer preferences.
Advantages of Prompt Tuning | Advantages of Retrieval Augmented Generation (RAG) |
---|---|
Enables quick customization of AI models Produces prompt and context-specific insights Reduces the need for extensive retraining Enhances adaptability in diverse business scenarios | Augments AI-generated outputs with relevant data Improves response accuracy and relevance Allows for the use of knowledge sources Extracts meaningful insights from complex data sets |
The Rise of Responsible AI
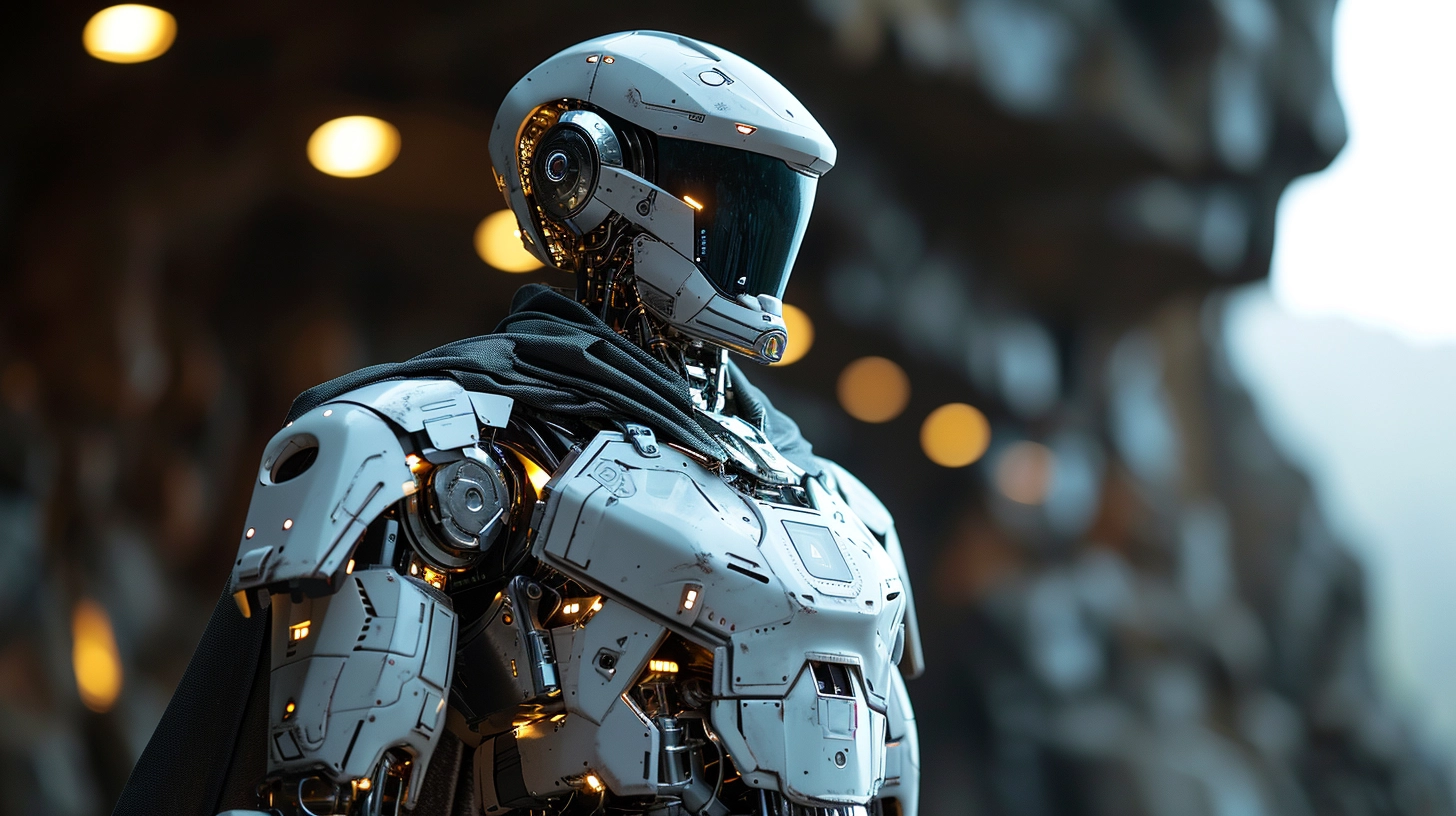
In 2024, businesses will experience a significant shift towards the ethical and responsible use of AI. With a growing emphasis on data ethics and algorithmic accountability, organizations will prioritize practices that protect privacy, ensure fairness, reduce bias, and enhance diversity and inclusion. This paradigm shift is not only driven by ethical concerns but also by the emergence of new legislative pressures.
Proposed legislation, such as the Algorithmic Accountability Act in the U.S. and the EU AI Act in the European Union, will require businesses to critically assess and report the impact of their automated decision systems. This regulatory push aims to hold companies accountable for the ethical implications of their AI technologies and brings attention to the need for data quality, privacy, security, and explainability of AI systems.
Responsible AI practices will become a cornerstone of technological advancement and application, safeguarding public trust and enabling businesses to gain a competitive advantage. By adhering to ethical standards and embracing transparency, organizations can foster trust among consumers, regulators, and the wider society.
Ethical AI practices are not just a legal obligation; they are a means to build a sustainable future that benefits everyone. It is our responsibility as AI practitioners to ensure that our algorithms uphold integrity, fairness, and respect for human values.
The Importance of Ethical Practices
Ethical practices in AI are vital for several reasons:
- Protecting privacy: Respecting individuals’ rights to privacy is crucial in an AI-powered world. Organizations must implement robust privacy measures to safeguard sensitive data and ensure compliance with relevant regulations, such as the General Data Protection Regulation (GDPR).
- Reducing bias: AI systems can inherit biases from the data used to train them, leading to discriminatory outcomes. By actively addressing bias during the development and deployment of AI models, businesses can promote fairness and equal opportunities.
- Ensuring algorithmic accountability: Transparent and explainable AI systems enable stakeholders to understand how automated decisions are made and challenge potential biases or erroneous outcomes. Algorithmic accountability promotes trust, comprehension, and facilitates meaningful dialogues between organizations and their users.
- Enhancing data quality: By prioritizing data quality and adopting reliable data collection and management practices, businesses can ensure the accuracy and reliability of their AI systems, leading to better decision-making and actionable insights.
- Addressing security concerns: Protecting AI systems from vulnerabilities is essential to prevent malicious attacks or unauthorized access to sensitive information. Robust security measures are necessary to uphold trust and maintain the integrity of AI technologies.
By embracing responsible AI practices, businesses can not only comply with emerging regulations but also build trust, foster innovation, and drive sustained success in an increasingly AI-driven world.
Unlock the Future: Harnessing AI Predictive Analytics Services
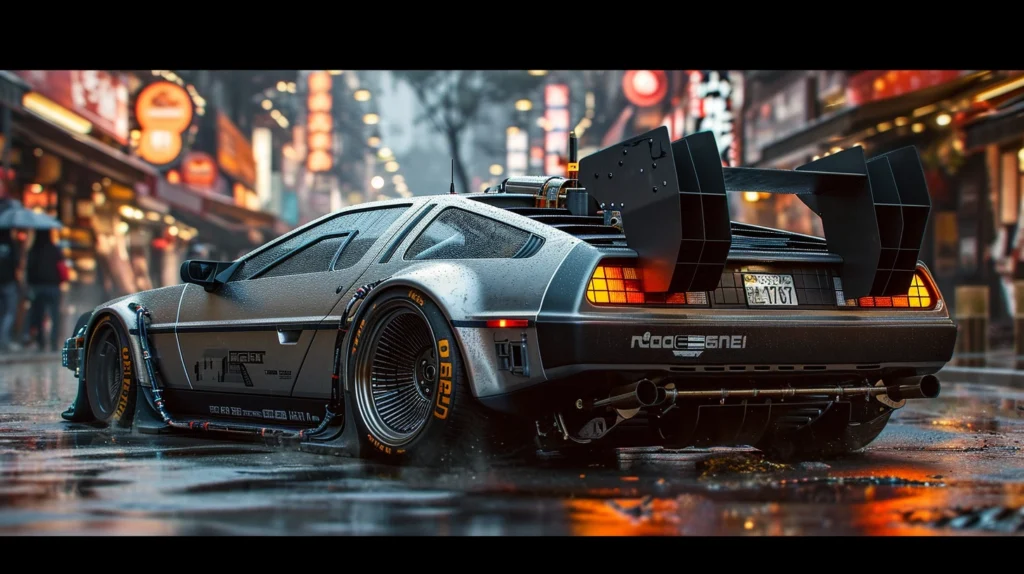
In the world of business, the fusion of AI and big data analytics is revolutionizing how companies operate. Cutting-edge predictive analytics software such as Tableau, Power BI, and Microsoft Azure Machine Learning are reshaping the landscape.
These tools, including others like Polymer, Julius AI, and DataRobot, offer a myriad of functionalities. From transforming raw data into structured, usable formats to deploying deep learning for nuanced insights, they’re pivotal in predicting customer behavior and guiding business strategies.
Predictive Analytics in Business: A Game Changer
Utilizing predictive analytics model, companies are not just analyzing data; they’re foreseeing the future. This technology enables businesses to:
- Visualize Complex Data: With tools like Sisense and Kanaries RATH, complex information is simplified, uncovering patterns and trends vital for business decisions.
- Transform Data for Insights: Data transformation capabilities structure and preprocess data, prepping it for intricate analysis.
- Extract Meaning from Text: AI-driven text analysis digs deep into customer reviews and social media comments, offering a goldmine of customer insights.
- Predictive Maintenance and Decision Making: Machine learning algorithms in tools like H2O.ai facilitate predictive maintenance, bolstering efficiency and foresight.
Custom Solutions for Diverse Needs
Businesses today need custom predictive analytics solutions tailored to their unique challenges. Financial services, marketing, or manufacturing, predictive analytics consulting services provide bespoke solutions. These include:
- Predictive Models for Customer Behavior: By leveraging historical data, businesses can predict customer needs and preferences, enhancing their products or services.
- Data Science and Machine Learning Integration: Integrating data science analysis with machine learning, companies can transform their data into actionable insights.
- Security and Efficiency: AI provides not only insights but also ensures data security, a critical aspect in today’s digital age.
The Future is Predictive
Predictive analytics tools, powered by AI, are not just tools but catalysts for change. They offer businesses the ability to use AI and historical data to make predictions, tailor their services, and stay ahead in the competitive market.
By developing predictive models and employing data science and machine learning, companies can make informed, data-driven decisions that drive growth and success.
Starting a Side Hustle in AI Predictive Analytics
Are you looking for a profitable side hustle that leverages your data analysis skills and keeps you on the cutting edge of technology? Consider starting a business in AI predictive analytics. Here’s how to get started:
What is AI Predictive Analytics?
AI predictive analytics uses machine learning algorithms to analyze historical data and identify patterns, trends, and insights. It enables businesses to make informed decisions by predicting future outcomes based on current data. (Source: Gartner)
Why Start a Side Hustle in AI Predictive Analytics?
- High Demand:Â According to Grand View Research, the global predictive analytics market size was valued at USD 11.2 billion in 2019 and is expected to grow at a compound annual growth rate (CAGR) of 14.1% from 2020 to 2027.
- Versatile Applications:Â AI predictive analytics can be applied to various industries, including healthcare, finance, retail, and marketing.
- Lucrative:Â As a side hustle, you can offer your services on a project basis or as a consultant, charging hourly rates or project fees.
Getting Started
- Learn the Basics:Â Familiarize yourself with machine learning algorithms, data mining techniques, and data visualization tools. Consider taking online courses or earning a certification.
- Build a Portfolio:Â Apply your skills to real-world projects, even if they’re small or for friends and family. This will help you build a portfolio to showcase your expertise.
- Network:Â Connect with other professionals in the field, attend industry events, and join online communities. Building a network can lead to new opportunities and collaborations.
- Market Your Services:Â Create a website or LinkedIn profile showcasing your skills and services. Utilize social media and other marketing channels to reach potential clients.
- Price Your Services:Â Determine your hourly rate or project fees based on your experience, expertise, and market demand. Be sure to consider your overhead costs, such as software and hardware expenses.
Tools and Resources
- Data Visualization Tools:Â Tableau, Power BI, and QlikView are popular data visualization tools that can help you create compelling data stories.
- Machine Learning Libraries:Â Scikit-learn, TensorFlow, and Keras are popular machine learning libraries that can help you build predictive models.
- Cloud Platforms:Â AWS, Microsoft Azure, and Google Cloud Platform offer various AI and machine learning services that can help you process and analyze large datasets.
Conclusion
Starting a side hustle in AI predictive analytics can be a rewarding and profitable venture. With the high demand for predictive analytics and the versatility of its applications, there’s no shortage of opportunities. By learning the basics, building a portfolio, networking, marketing your services, and utilizing the right tools and resources, you can establish a successful side hustle in this exciting field.
Answering FAQ’s: AI in Predictive Analytics Services
How is AI used in predictive analytics?
AI in predictive analytics involves the use of algorithms to analyze data and identify patterns. This enables predictions about future events or trends based on current and past information. In the context of event planning, AI can analyze vast amounts of attendee data, social media posts, and feedback to predict future attendee behavior, preferences, and engagement levels. This helps event planners optimize the attendee experience, tailor event schedules, and enhance overall event performance.
What function does AI service in predictive analysis?
AI serves several crucial functions in predictive analysis. It automates the analysis of large data sets, efficiently identifying patterns and making informed predictions. For event organizers, this means leveraging AI to anticipate future trends in the events industry, determine the most effective marketing efforts, and predict attendee engagement. AI’s ability to process information rapidly allows event professionals to gain real-time insights and make data-driven decisions for future events.
What is AI-powered analytics?
AI-powered analytics refers to the application of machine learning techniques in data analysis to uncover insights, identify new patterns, and discover relationships in data. In the events industry, AI-powered analytics can be used to analyze attendee behavior, preferences, and demographics. This analysis helps in creating more personalized and engaging experiences, from tailoring event content to optimizing the planning process for hybrid or virtual events.
How can AI be used in data analytics?
AI can be used in data analytics to process and analyze large sets of data more efficiently than human intelligence. In the context of event planning, AI can be employed to analyze feedback from various sources, including social media platforms and event apps. This helps in understanding attendee preferences and engagement levels, aiding in the creation of more successful and unforgettable events. Additionally, AI-powered tools like virtual assistants and chatbots can enhance customer service and attendee experience by providing real-time assistance and information.
References
- Predictive analytics: a review of trends and techniques
- From analytics to artificial intelligence
- Data mining and predictive analytics applications for the delivery of healthcare services: a systematic literature review
- Protecting your patients’ interests in the era of big data, artificial intelligence, and predictive analytics
- Machine learning and AI in business intelligence: Trends and opportunities